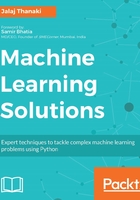
Best approach
As mentioned in the previous section, in this iteration, we will focus on feature transformation as well as implementing a voting classifier that will use the AdaBoost and GradientBoosting classifiers. Hopefully, by using this approach, we will get the best ROC-AUC score on the validation dataset as well as the real testing dataset. This is the best possible approach in order to generate the best result. If you have any creative solutions, you can also try them as well. Now we will jump to the implementation part.
Implementing the best approach
Here, we will implement the following techniques:
- Log transformation of features
- Voting-based ensemble model
Let's implement feature transformation first.
Log transformation of features
We will apply log transformation to our training dataset. The reason behind this is that we have some attributes that are very skewed and some data attributes that have values that are more spread out in nature. So, we will be taking the natural log of one plus the input feature array. You can refer to the code snippet shown in the following figure:

Figure 1.63: Code snippet for log(p+1) transformation of features.
I have also tested the ROC-AUC accuracy on the validation dataset, which gives us a minor change in accuracy.
Voting-based ensemble ML model
In this section, we will use a voting-based ensemble classifier. The scikit-learn library already has a module available for this. So, we implement a voting-based ML model for both untransformed features as well as transformed features. Let's see which version scores better on the validation dataset. You can refer to the code snippet given in the following figure:

Figure 1.64: Code snippet for a voting based ensemble classifier
Here, we are using two parameters: weight 2 for GradientBoosting and 1 for the AdaBoost algorithm. I have also set the voting parameter as soft so classifiers can be more collaborative.
We are almost done with trying out our best approach using a voting mechanism. In the next section, we will run our ML model on a real testing dataset. So let's do some real testing!
Running ML models on real test data
Here, we will be testing the accuracy of a voting-based ML model on our testing dataset. In the first iteration, we are not going to take log transformation for the test dataset, and in the second iteration, we are going to take log transformation for the test dataset. In both cases, we will generate the probability for the target class. Here, we are generating probability because we want to know how much of a chance there is of a particular person defaulting on their loan in the next 2 years. We will save the predicted probability in a csv
file.
You can see the code for performing testing in the following figure:

Figure 1.65: Code snippet for testing
If you can see Figure 1.64 then you come to know that here, we have achieved 86% accuracy. This score is by far the most efficient accuracy as per industry standards.